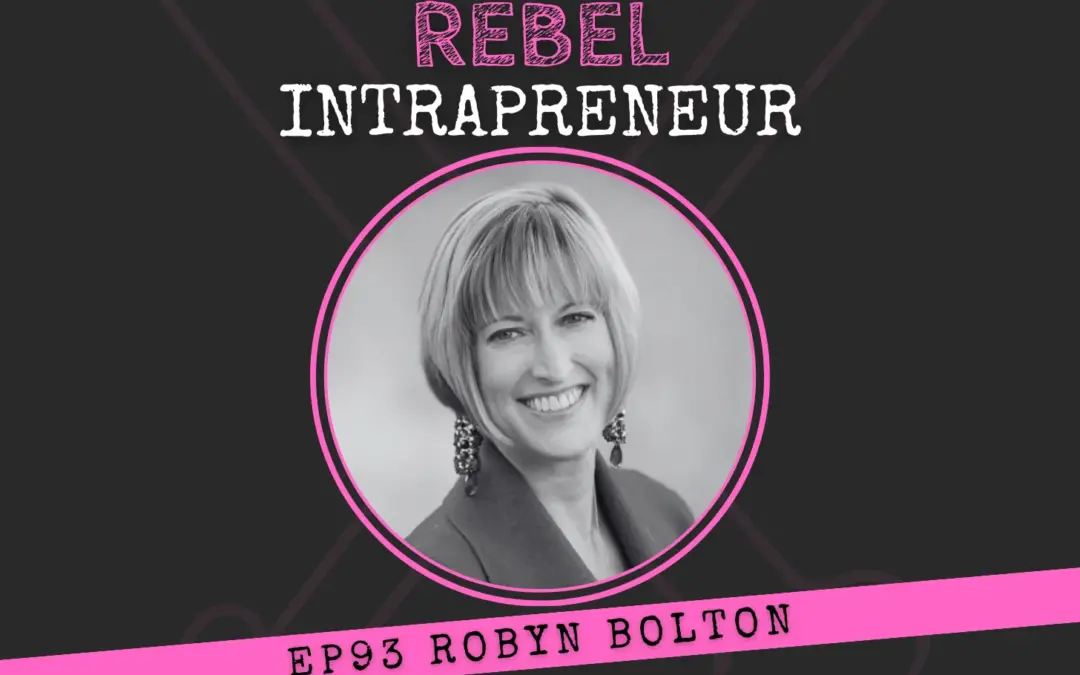
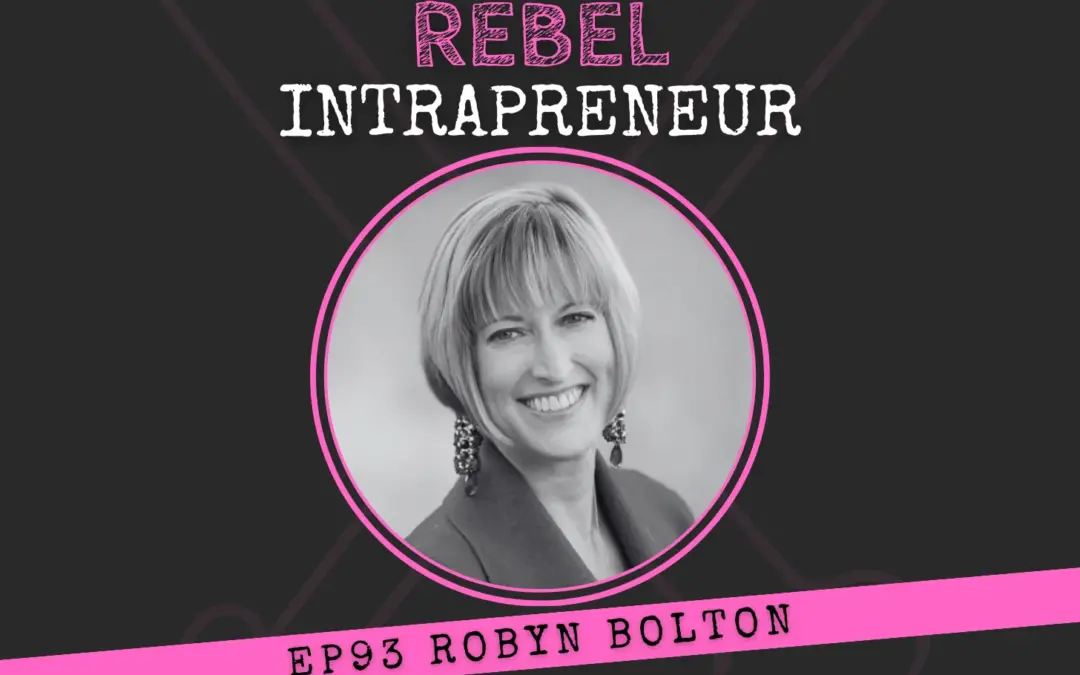
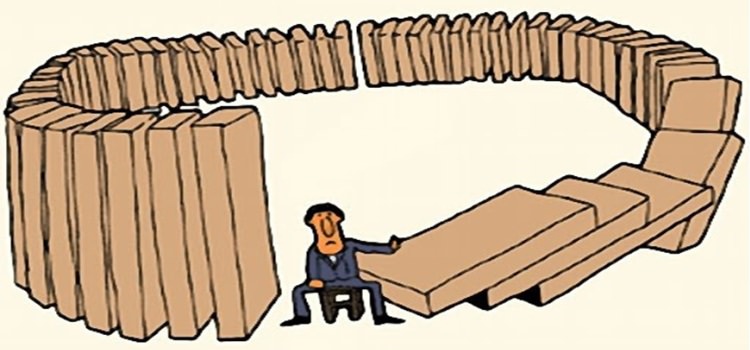
The 93% Rule: How to Predict Unintended Consequences
Unintended consequences often catch us off guard despite their predictability. The moment they occur, we gasp in shock, shake our heads, and look at each other in wide-eyed horror at this thing that just happened that we could never ever ever have anticipated.
Yet, when (if) we do an After-Action Review, we often realize that these consequences were not entirely unforeseeable. In fact, had we anticipated them, we might have made different decisions.
The Unintended Consequences of Spreadsheets
In 1800 BCE, ancient Babylonians started recording data by scratching grids and columns onto clay tablets, and the spreadsheet was born. Over the millennia, we went from clay tablets to papyrus to parchment and then paper.
Fast forward to 1963 when R. Brian Walsh of Marquette University ported the Business Computer Language (BCL) program to an IBM 7040, and electronic spreadsheets became a reality. The introduction of VisiCalc by Apple in 1979 revolutionized spreadsheet capabilities, followed by Lotus 123 and Microsoft Excel. Today, spreadsheets are ubiquitous in education, business operations, financial markets, budgeting, and even personal inventories.
Unintended yet predictable consequences
While spreadsheets have undoubtedly enhanced efficiency and accuracy compared to traditional methods like clay tablets or hand-drawn tables on parchment, their ease of use has inadvertently led to complacency.
We stopped engaging in a multi-millennial habit of discussing, debating, and deciding before making a spreadsheet. We started flippantly asking people to create spreadsheets and providing little, if any, guidance because “it’s easy to make changes and run scenarios.”
This shift resulted in a reliance on automated models and a lack of shared assumptions or analytical rigor in decision-making processes.
Of course, these behaviors were never intended. They were, however, very predictable.
93% of Human Behavior is predictable.
Research spanning disciplines as varied as network scientists, anthropology, neuropsychology, and paleontology shines a light on how truly predictable we are.
Emotions before Reason: Ask someone if they make decisions based on their motivations, aspirations, and fears and use data to justify the decisions, and they’ll tell you no. Ask them the last time someone else made a decision that “made no sense,” and you’ll listen to a long list of examples.
Small gains now are better than big gains later: Thoughtfully planning before using solutions like spreadsheets, word processing, email, and instant messaging could save us time at work and help us get home 30 minutes earlier or work a few hours less on the weekend. But saving a few seconds now by brain-dumping into Word, setting up a “flexible” spreadsheet, and firing off a text feels much better.
Confidence > Realism: We’ve all been in meetings where the loudest voice or the most senior person’s opinion carried the day. As we follow their lead, we ignore signs that we’re wrong and explain away unexpected and foreboding outcomes until we either wake up to our mistakes or adjust to our new circumstances.
Predict the 93%. Create for the 7%
Acknowledging the predictability of human behavior is not an endorsement of stereotypes but a recognition of our innate cognitive processes. By incorporating this understanding into design, innovation, and decision-making processes, we better anticipate potential outcomes and mitigate unintended consequences.
While 93% of human behavior may follow predictable patterns rooted in evolutionary instincts, focusing on the remaining 7% allows for the exploration of unique behaviors and novel solutions. By embracing both aspects of human nature, we can navigate challenges more effectively and anticipate a broader range of outcomes in our endeavors, leading to informed decision-making and value creation.
Now, if I could only get Excel to stop auto-converting numbers into date/time format.
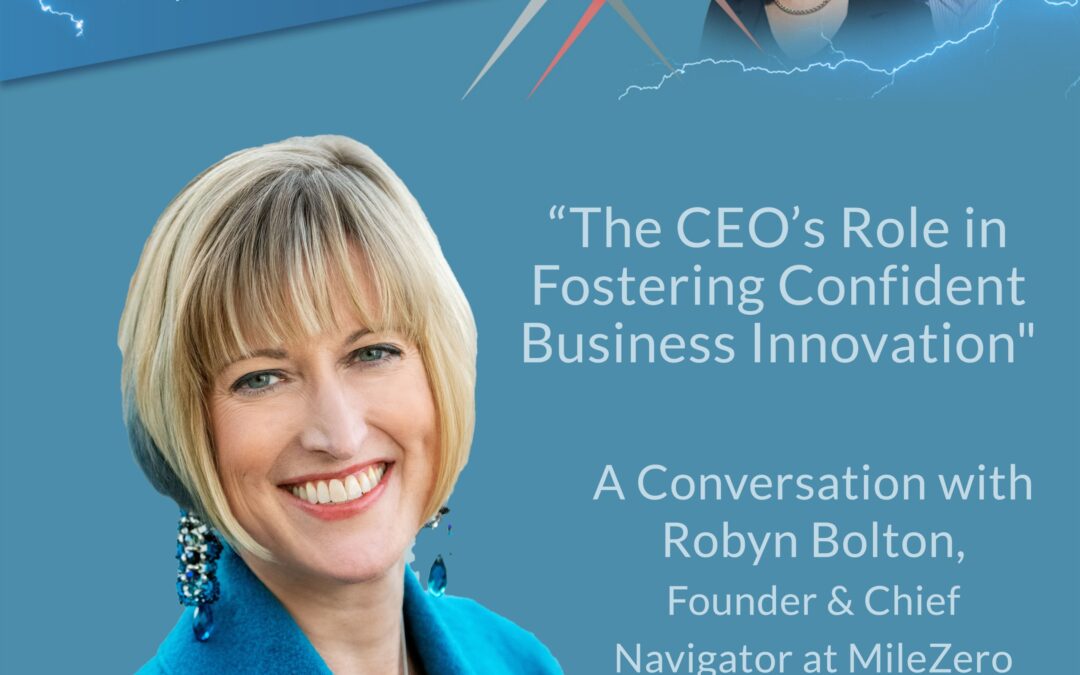
Growth Igniters® Radio
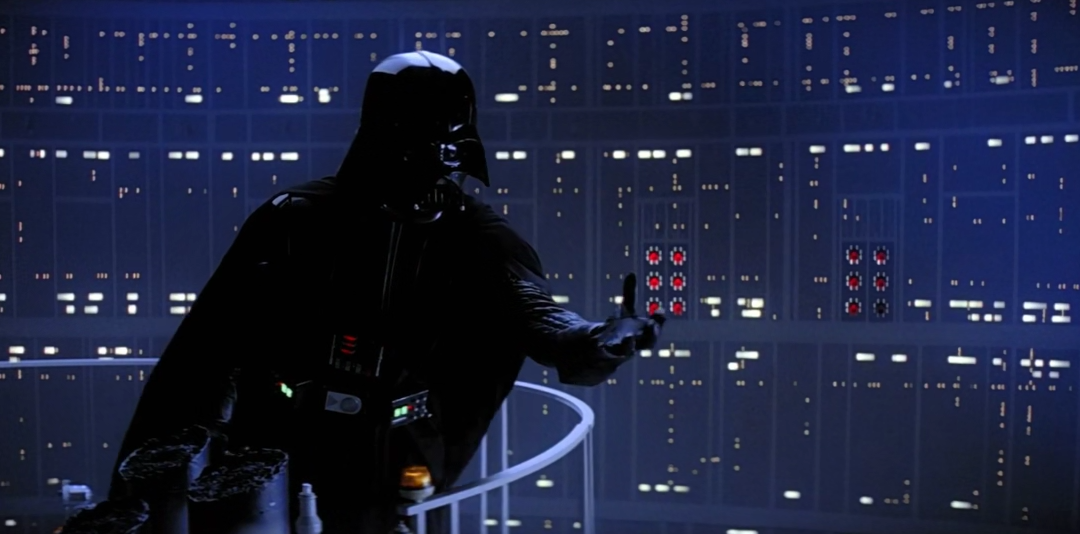
The Positive Power of ‘Negative’ Emotions in Driving Change
You want to make life better for others. This desire is reflected in the optimism and positivity of your language – create value, love the problem, and delight the customer. But making life better requires change, and, as the adage goes, “People want change, but they don’t want to be changed.”
You are confident that the solution you created will make life better and that the change people need to make is quite small and painless, well worth the dramatic improvement you offer. Yet they resist. No amount of explaining, showing, convincing, or cajoling changes their mind. What else can you do?
To quote Darth Vader, “Give yourself to the Dark Side. It is the only way to save your friends.”
“If only you knew the power of the Dark Side…”
The Dark Side is populated by “negative” emotions like anger, fear, and frustration, which are incredibly powerful.
Consider that:
- Negative events are remembered more accurately and in greater detail than positive events
- Six positive comments are required to offset the effect of one negative comment
- 60% of people make purchases fueled by their fear of missing out (FOMO)
Unfortunately, these are also some of the first emotions experienced when confronting change.
Change requires people to let go of what they know in exchange for the promise of something better. This immediately triggers Loss Aversion, the cognitive bias in which the pain of losing is psychologically twice as powerful as the pleasure of gaining.
As a result, people won’t let go of what they know until the pain of holding on becomes unbearable. When you point out the problems and pain of the current situation, you help people understand and experience the unbearableness of the current situation.
“Anger, fear, aggression; the Dark Side of the Force are they”
Not every “negative” emotion elicits the same behavior, so carefully choose the one to tap into.
Fear motivates people to seek safety, which can be good if your solution truly offers a safer alternative. It’s a motivator used well by companies such as Volvo, SimpliSafe, and Graco. But lean on it too much, and people may feel overwhelmed and remain frozen to the status quo.
Anger motivates people to take risks, which can be good when the change requires bold decisions and dogged persistence. It can be great when it bonds people together to achieve a shared goal or protect a common value. Apple used this emotion to brilliant effect in its famous “1984” commercial announcing the launch of Macintosh. But incite too much anger, and things can get broken and not in a helpful way like Apple’s ad.
Frustration, one of the emotions that often drives aggression, is anger’s polite little sister. When people feel frustrated, they’re likely to act, persistently pursue solutions, and creatively approach and overcome obstacles. But if the change is big, feels scary, and puts their sense of self at risk, frustration isn’t powerful enough to convince people to let go of the old and embrace the new.
“If you start down the dark path, forever will it dominate your destiny.”
Yoda is incredibly wise, but he gets this one wrong. Using the Dark Side to speak to people’s “negative” emotions doesn’t doom you to a life or career of fear-mongering or inciting violence. Start here, don’t stay here.
Multiple research studies show that positive emotions, like hope and joy, are more powerful than negative ones in maintaining motivation and even enable more creative thinking and problem-solving. By speaking to both negative and positive emotions, the Dark Side and the Light, you enable change by giving people a reason to let go of the past and a future worth reaching for.
When people stop resisting and start reaching to the future you’re offering, change happens, and you realize that Yoda was right, “Luminous beings are we, not this crude matter.”
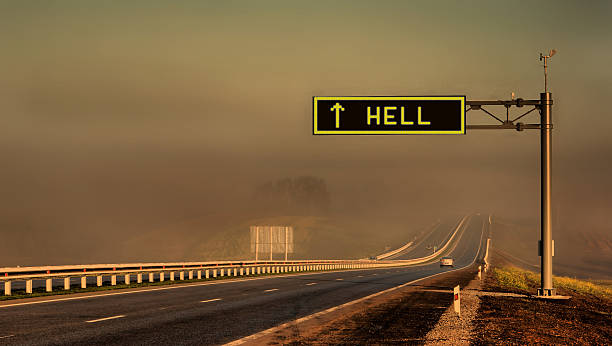
New Data Shows that Good Intentions Pave the Way to Innovation Hell
The road to hell is paved with good intentions, and nowhere is that more true than in innovation.
That’s one of the insights I took away from InnoLead’s Q1 report on corporate innovation priorities. The report is an eye-opening look at the impact of AI on corporate innovation as experienced by corporate entrepreneurs themselves. But before deep diving into that topic, the report’s authors shared intriguing data about member companies’ innovation structure, leadership engagement, organizational connections, and results. Nestled amongst the charts were several that, when taken together, got my Spidey senses tingling.
61.0% of innovation teams are “directly under a high-visibility leader with a broad company focus.”
This is great because innovation needs senior leaders’ support and active engagement to survive, let alone survive for long enough to produce meaningful results. Add this to the fact that 45% of senior leadership teams frequently discuss the “progress and value of the innovation program,” and all signs point to innovation as a strategic priority.
But (you knew there was a but, didn’t you)…
If “broad company focus” means “no P&L responsibility,” we have a problem. In every for-profit company I’ve worked for and with, people with P&L responsibility have greater power, influence, and access to resources than people without a P&L. This division may not feel fair, but it makes sense – the people who bring in profit and revenue will always be more influential than people who represent “cost centers.”
You can see the impact of P&L owners who are, understandably, focused entirely on delivering short-term results throughout the report – 75% of companies have shifted their focus more towards near-term priorities, and 61% shifted their innovation portfolio away from Horizon 3 (also known as radical, breakthrough, or disruptive innovation).
As for all those discussions, it’d be great if they focused on walking the talk of innovation. But suppose it’s only innovation platitudes or, worse, questioning innovation’s ROI. That doesn’t bode well for the “high-visibility leader with broad company focus,” the innovation team, or the company’s culture.
71.2% of innovation teams’ customers or business partners are unaware of the team’s existence, don’t engage, or engage only occasionally.
Welcome to Innovation Island! Where the cool people work on cool things in cool offices while all you drones slave away doing the same thing you’ve always done and making the money that pays for the cool people to do cool things in their cool offices.
I’m sure this isn’t the message the innovation team intends to send, but it’s the one received by most organizations.
When arguing for Innovation Island, managers often point to the organizational antibodies likely to swarm and kill H3/radical/breakthrough innovation and even some H2/adjacent innovations. They’re right, and those innovations must be “protected.” But not every innovation needs protection. H2 and certainly H1 innovations, where most portfolios are now, should be shared with the core business because the core business will eventually run them.
The bigger problem, in my opinion, is that innovation teams don’t seem to be reaching out to others in the organization. Like the P&L owners they report to, people in the core business are busy running the business and generating revenue. Very few have the time or energy to seek out the innovation team to discuss and explore innovation. Companies that want to build a culture of innovation need to turn their innovators into evangelists, not residents of an island connected to the mainland by a single drawbridge.
23.4% of innovation teams are considered outsiders or actively undermined by other functions and business units.
This may not sound bad, but add to it the 55.0% that are “somewhat integrated with occasional collaboration” with other departments and business units, and you may be tempted to believe that Innovation Island would be wise to invest in a surface-to-air missile defense system.
Sadly, this perception of the innovation team as “The Others” isn’t surprising when considering that the most important tactic for building a relationship between innovation and the functions or business units is already having strong relationships and interpersonal trust (75.3% of respondents). The least effective (4.7% of respondents) is “writing down shared objectives and expectations.” So, no, the email you sent is not enough to win friends and influence people.
Bottom line
Well-intended companies appoint a senior executive to lead the innovation team because they’ve been told that doing so is powerful proof that innovation is a strategic priority. They hire outsiders to inject new thinking into the organization because they know that “what got you here won’t get you there.” They cordon the team and their work off from the rest of the organization because they read that separation is essential to preserving innovation’s disruptive nature.
But if the senior executive doesn’t have the organizational power and influence that comes with P&L ownership, the team doesn’t have strong personal relationships with others in the business, and other functions and business units don’t know the team exists or how to interact with it, innovation will go nowhere.
But that’s better than where it could go.